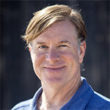
Where Matters: The Significance of Location in Vehicle Telemetry Data Analysis
The proliferation of sensors in automobiles has ushered in a new era of data-rich vehicles, offering immense benefits in terms of analyzing this wealth of information and creating data-driven products and features. Modern connected vehicles are equipped with a multitude of sensors, including those for engine performance, safety, navigation, and connectivity. These sensors continuously generate a vast array of data, encompassing everything from speed and fuel consumption to environmental conditions and driver behavior. This data, when harnessed effectively, enables automakers and tech companies to develop innovative products and features that enhance safety, convenience, and overall driving experience.
By analyzing this data, insights can be gleaned about vehicle performance, predictive maintenance, energy efficiency, and even real-time traffic conditions, leading to the development of intelligent features like adaptive cruise control, predictive maintenance alerts, and advanced driver-assistance systems (ADAS). Moreover, the potential extends beyond the vehicle itself, as this data can be leveraged to create new services, such as usage-based insurance, fleet management solutions, and smart city initiatives that rely on traffic and environmental data from connected vehicles. The proliferation of sensors in automobiles has given rise to a data-driven automotive ecosystem that promises to revolutionize how we interact with and benefit from our vehicles.
Vehicle sensor data is characterized by its sheer volume and fast-moving nature, making it a dynamic and high-frequency data source. It comprises a continuous stream of readings, each associated with a timestamp and geospatial coordinates in the form of longitude and latitude. The timestamp provides a chronological context, allowing for the tracking of events over time, while the geographical coordinates offer critical location-based insights. This combination of time and location data is essential for understanding not only what is happening with a vehicle but also where and when it is occurring.
For example, consider this abstracted view of a suspension reading. The camber of the left front tire is one of many suspension readings, along with caster, toe, and thrust angle. The measurement changes and is recorded every second, along with the changing location of the vehicle. A simple use case on this data would be to detect potholes, their depth, and the range of speeds in which the potholes were hit by drivers. Such information could be used to avoid these hazards and provide municipalities with insights to help with prioritizing road maintenance.
Yet, many auto manufacturers are currently storing and analyzing vehicle telemetry data using tools that primarily focus on time-series data, often lacking advanced spatio-temporal capabilities such as InfluxDb or Spark. InfluxDB, a time-series database, has traditionally been used by some auto manufacturers despite disclaimers that the “geo package is experimental and subject to change at any time” and “risky.” Spark currently offers only rudimentary spatio-temporal capabilities, and efforts within the Apache ecosystem, such as GeoMesa and Sedona, aimed at addressing these limitations have not yet delivered comprehensive solutions.
Using a time-series database for vehicle telematics data that is inherently both time-series and spatial in nature can result in a range of significant pitfalls. One of the most glaring issues is the loss of crucial information related to the location of vehicles. Telematics data often includes GPS coordinates, which are essential for tracking the precise whereabouts of vehicles, monitoring routes, and ensuring driver safety. In a time-series-only database, this spatial information is either completely discarded or treated in a primitive manner, reducing the ability to gain insights into the geospatial aspects of vehicle operations. This can severely limit the effectiveness of telematics applications, such as route optimization, geofencing, and real-time monitoring, all of which heavily rely on spatial data.
Furthermore, relying solely on a time-series database for telematics neglects the holistic nature of the data. Failing to incorporate the spatial dimension of the data means that critical insights, such as identifying the exact location of accidents or the proximity of vehicles to certain landmarks or destinations, are compromised. This can have far-reaching implications for fleet management, insurance assessments, and regulatory compliance, as these functions often require a nuanced understanding of the interplay between time and location. To harness the full potential of vehicle telematics data, a database should effectively capture and integrate both time-series and spatial elements, ensuring that no critical information is overlooked or underutilized.
Context matters, and when it comes to vehicle telemetry data, contextual data is fused not on primary keys but on spatial and temporal dimensions. Supporting temporal and geo-joins with telemetry data is crucial for unlocking a deeper understanding of complex systems and driving data-driven insights. For instance, combining vehicle telemetry data with road network information enables precise route optimization, real-time traffic management, and accident analysis. Similarly, integrating telemetry data with weather data empowers applications like dynamic weather-influenced routing, enhancing safety and efficiency. These temporal and geo-joins allow for a comprehensive analysis of data, facilitating the development of intelligent solutions that can significantly impact transportation, logistics, and safety-related decision-making.
Ford has emerged as a leader in the realm of Advanced Driver Assistance Systems (ADAS) through its groundbreaking BlueCruise system. A recent Consumer Reports article ranked Ford #1 in the industry (including Tesla that comes as a shock to many). While there are many facets contributing to this achievement, one standout reason is Ford’s innovative approach and unwavering dedication to constructing a cutting-edge data platform that excels in real-time location analytics. By harnessing both the temporal and spatial nature of vehicle data in a comprehensive and intelligent manner, Ford has managed to create a pioneering ADAS system that has set new standards in the automotive industry, making roads safer and driving experiences more comfortable for their customers.
Kinetica was designed from the ground up to excel at spatio-temporal analytics. The database platform offers a comprehensive set of tools that cater to the intricacies of vehicle telemetry data analysis. Kinetica is also at the forefront of leveraging Generative AI (Gen AI) for vehicle telematics by harnessing large language models to generate complex analytic code in plain english (aka NL2SQL) tuned for vehicle telemetry questions. Kinetica is also enabling real-time vector search on vehicle telematics to uncover previously hidden patterns leading to enhancements in safety, efficiency, and various other aspects of automotive technology.
Making Sense of Sensor Data
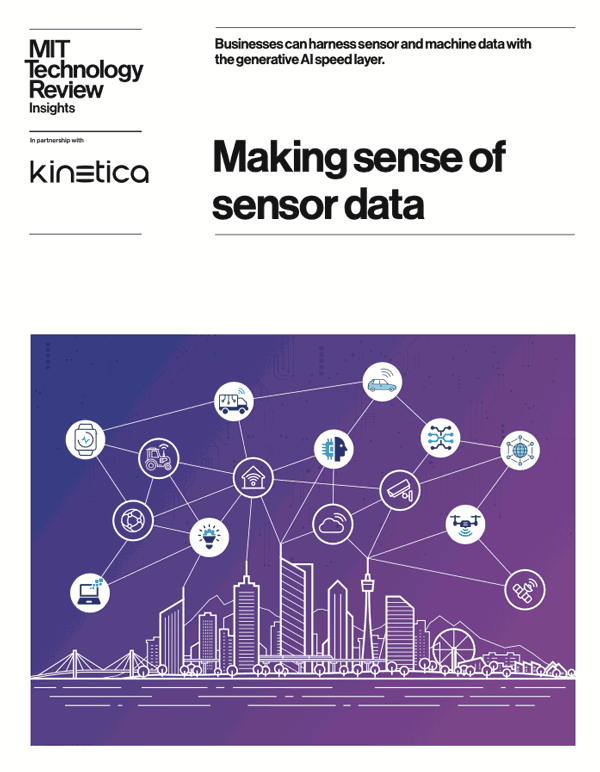