You Have An Extreme Data Problem! You Just Don’t Know It Yet.
As the importance of data has grown over the years, the avenues to learn how to store it, manage it, process it, and analyze it have grown as well. Conferences related to data and analytics continue to grow every year. In fact, March is always quite a busy month with several big events, including the Gartner Data & Analytics Summit and the O’Reilly Strata Data Conference. Attending these events generates meaningful conversations with technologists and tech-savvy business leaders, including business analysts, data scientists, product managers, data analytics leaders, and more.
The conversations vary widely from how to help speed up analysis on legacy relational databases, to understanding why streaming data analysis is important, to how to start to see a return on AI investments, to great t-shirt taglines. If you were a fly on the wall for these conversations, a pattern would start to emerge (leaving out the taglines, of course).
Most data analytics experts and business analysts are starting to feel like data is overwhelming them and their infrastructure, and, more importantly, that there are more insights in their data than they are able to take advantage of.
The data scientists, on the other hand, seem to spend a lot of time improving machine learning and deep learning models for their domains. But it’s the Wild West when it comes to how they access and manage their data, and most of the models that are built never step outside the research lab into a production environment.
Across industries, data and analytics–including the use of big data technologies–have been helping businesses make informed decisions. Over the years, organizations have moved from data-validated (using data to justify previous business decisions) to data-informed (using data to proactively make business decisions).
But the dimensions of data have fundamentally changed. Although the volume, variety, and velocity of data has been increasing over the years, what businesses are now dealing with is the unpredictability of that data. Not only do they need to manage and analyze static data but as new data sources continue to emerge, tomorrow they may be required to analyze streaming data as well.
Human-generated data and machine-generated data have very different characteristics. Data could be structured or unstructured. Data may be long-lived or perishable. Businesses are struggling to deal with unpredictable data.
Given this unpredictable data environment, the complexity of data analysis has increased exponentially. In finance, asset risk calculations are becoming harder, with hundreds of variables to simulate. In logistics, streaming data analysis for real-time fleet management and optimization is increasingly complex. In retail, challenges like micro-segmentation and micro-personalization mean solving a much more difficult data problem. In telecom, analyzing exploding network and device usage is getting more complicated every day.
It is clear to me that technologies built for the past–technologies built for only static, structured data or those optimized for a twenty-year-old hardware stack–are not going to keep up with solving these emerging extreme data problems. As the need to apply artificial intelligence to data increases, even distributed or scalable CPU-powered software will not be able to keep up. Technologies built with a combination of three core concepts together will be able to solve these complex data challenges: in-memory data management, GPU-based data processing, and distributed columnar data organization.
Need a primer on a GPU database? Read a quick overview here.
While you are thinking about the day-to-day challenges of processing your nightly batch data set, including ingesting, transforming, analyzing, and then finally deriving insights, think beyond. Think about what you are not seeing from your data; think about what benefits you can harvest with faster and deeper insights; think about the value of taking your trained machine learning models and operationalizing them; and think about what augmenting your classical data analytics workloads outside the lab would bring to the business.
You have an extreme data problem! You just don’t know it yet.
Editor’s Note: This article was originally published on Forbes on 4/23.
Making Sense of Sensor Data
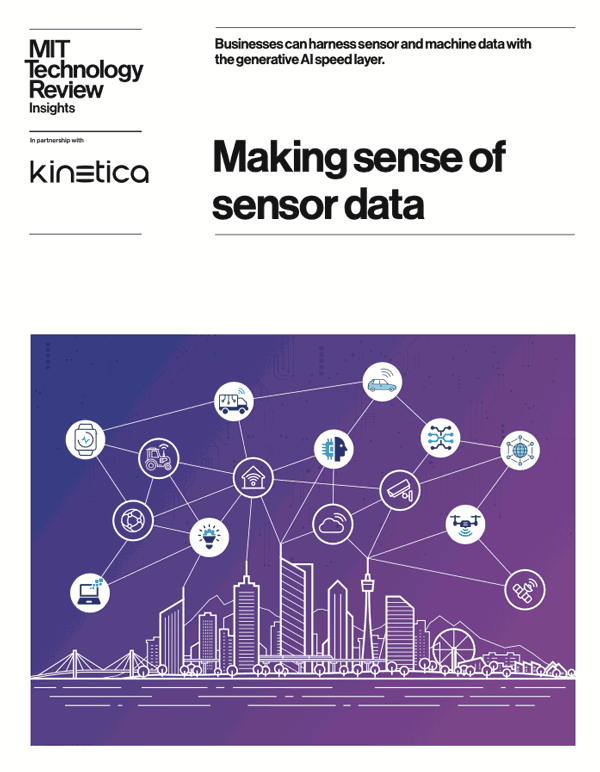