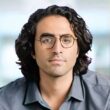
OpenAI’s Acquisition of Rockset: A Bellwether for the Future of Real-time Enterprise Analytics and Vector Search
The release of ChatGPT marked a significant shift in how people interact with technology by introducing a conversational mode of inquiry using natural language to surface insights. This trend is now extending to enterprise analytics, as evidenced by OpenAI’s acquisition of Rockset. The trend is clear: traditional BI tools and data science languages are giving way to natural language and conversational interfaces.
Real-time Multimodal Capabilities for AI Copilots
Kinetica’s database engine is uniquely suited for AI copilots because it excels in two critical areas: analytical range and low-latency responses. Conversational inquiries can lead to unpredictable and diverse types of queries. Kinetica supports a wide array of analytical tasks, including spatial, OLAP, graph, time series, and vector search, ensuring comprehensive analytical coverage required to support a conversation mode of inquiry.
Additionally, when dealing with enterprise-scale data, maintaining a conversational flow requires fast query responses. Kinetica’s architecture, leveraging modern CPUs and GPUs, guarantees high-speed processing, allowing for quick and seamless transitions from language to insight.
Furthermore, the speed with which data can be ingested into and extracted from Kinetica is exceptionally fast due to our distributed architecture. This ensures low data latency, complementing our fast query response times and making Kinetica an ideal platform for powering AI copilots.
From English to Insight: A Seamless Journey
An LLM does not have any context about your data. To generate the queries required to draw insights, it needs to be provided this information. Over the last year, Kinetica has introduced new capabilities that allow users to catalog their data, describing tables, columns, and relationships. When a user types in a question a Generative AI application will send Kinetica a request for a data context. Kinetica will dynamically construct this context in a way that respects enterprise security requirements down to the row and column level. This ensures that LLMs can frame queries correctly while guaranteeing that sensitive information is not exposed to the wrong user.
Kinetica also introduced advanced vector search capabilities that leverage GPU and CPU parallelization. This ensures that vector search queries benefit from the same high performance as other queries on Kinetica. We can ingest streams of vector embedding data and query them before indexing, reducing latency and allowing immediate query availability. This capability is essential for building generative AI applications that provide real-time insight as embeddings are generated.

Our customer are already seeing tremendous benefits
Our customers are already using the features described here to power AI copilots that decision makers use to query data in plain English. Our speed supports Gen. AI applications at enterprise scale, providing significant gains in the speed and ease of deriving insights—all without writing a single line of code.
OpenAI’s acquisition of Rockset highlights a significant trend: the growing importance of natural language interfaces for enterprise analytics. Kinetica is uniquely positioned to lead this transformation. With our powerful and versatile database engine, designed for the demands of modern AI applications, we are ready to empower enterprises to turn natural language queries into immediate, actionable insights. As we continue to innovate, our mission remains clear—to provide enterprises with the most cutting edge tools they need to leverage their data.
Making Sense of Sensor Data
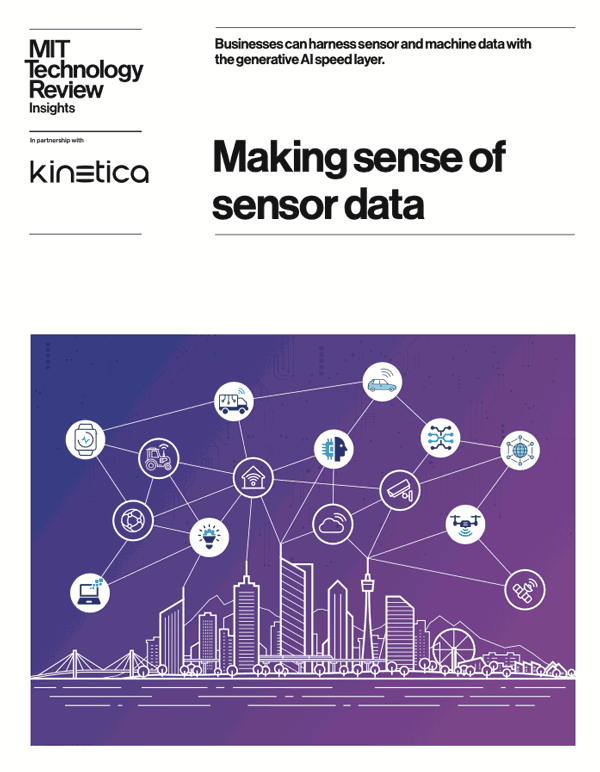